ML - Clustering#
Motivation#
World map showing country classifications as per the IMF (International Monetary Fund) and the UN (United Nations) (last updated 2022).
Blue: Developed countries
Orange: Developing countries
Red: Least developed countries
Gray: Data unavailable
Most commonly, the criteria for evaluating the degree of economic development are gross domestic product (GDP), gross national product (GNP), the per capita income, level of industrialization, amount of widespread infrastructure and general standard of living.
Question: Can we categorize countries based on these features without having labels from the beginning? Why are there only three categories?
Answer: Clustering! This is how we categorize elements without previous labels.
K-means#
Input: Feature matrix \(X\) and a hyper-parameter \(K\) that determine the number of clusters.
Output: Cluster centroids (\(u_k\), \(k=1, 2, \ldots, K\)) and labels for each row of \(X\) that indicate which cluster it belongs to.
This is a NP-hard problem (impossible to solve in polynomial time, the most difficult type of NP problem).
LLoyd Algorithm#
Compute the centroid of the cluster by averaging the positions of the elements currently in the cluster.
Update cluster label of the elements using the closest distance to each centroid.
In this case, one video is worth more than a thousand pictures.
from IPython.display import YouTubeVideo
YouTubeVideo("5I3Ei69I40s")
Implementation#
import numpy as np
import pandas as pd
from pathlib import Path
filepath = "https://raw.githubusercontent.com/aoguedao/neural-computing-book/main/data/gapminder.csv"
# filepath = Path().resolve().parent / "data" / "gapminder.csv" # If you are running locally
data = pd.read_csv(filepath, usecols=[1, 5, 6])
data.head()
country | life_exp | gdp_cap | |
---|---|---|---|
0 | Afghanistan | 43.828 | 974.580338 |
1 | Albania | 76.423 | 5937.029526 |
2 | Algeria | 72.301 | 6223.367465 |
3 | Angola | 42.731 | 4797.231267 |
4 | Argentina | 75.320 | 12779.379640 |
from sklearn.cluster import KMeans
K = 3
kmeans = KMeans(n_clusters=K)
kmeans.fit(data.drop(columns="country"))
/opt/hostedtoolcache/Python/3.8.16/x64/lib/python3.8/site-packages/sklearn/cluster/_kmeans.py:870: FutureWarning: The default value of `n_init` will change from 10 to 'auto' in 1.4. Set the value of `n_init` explicitly to suppress the warning
warnings.warn(
KMeans(n_clusters=3)In a Jupyter environment, please rerun this cell to show the HTML representation or trust the notebook.
On GitHub, the HTML representation is unable to render, please try loading this page with nbviewer.org.
KMeans(n_clusters=3)
data["label"] = kmeans.labels_
data.head()
country | life_exp | gdp_cap | label | |
---|---|---|---|---|
0 | Afghanistan | 43.828 | 974.580338 | 2 |
1 | Albania | 76.423 | 5937.029526 | 2 |
2 | Algeria | 72.301 | 6223.367465 | 2 |
3 | Angola | 42.731 | 4797.231267 | 2 |
4 | Argentina | 75.320 | 12779.379640 | 0 |
data.query("label == 2")
country | life_exp | gdp_cap | label | |
---|---|---|---|---|
0 | Afghanistan | 43.828 | 974.580338 | 2 |
1 | Albania | 76.423 | 5937.029526 | 2 |
2 | Algeria | 72.301 | 6223.367465 | 2 |
3 | Angola | 42.731 | 4797.231267 | 2 |
8 | Bangladesh | 64.062 | 1391.253792 | 2 |
... | ... | ... | ... | ... |
137 | Vietnam | 74.249 | 2441.576404 | 2 |
138 | West Bank and Gaza | 73.422 | 3025.349798 | 2 |
139 | Yemen, Rep. | 62.698 | 2280.769906 | 2 |
140 | Zambia | 42.384 | 1271.211593 | 2 |
141 | Zimbabwe | 43.487 | 469.709298 | 2 |
80 rows × 4 columns
data.query("label == 1")
country | life_exp | gdp_cap | label | |
---|---|---|---|---|
5 | Australia | 81.235 | 34435.36744 | 1 |
6 | Austria | 79.829 | 36126.49270 | 1 |
7 | Bahrain | 75.635 | 29796.04834 | 1 |
9 | Belgium | 79.441 | 33692.60508 | 1 |
20 | Canada | 80.653 | 36319.23501 | 1 |
34 | Denmark | 78.332 | 35278.41874 | 1 |
43 | Finland | 79.313 | 33207.08440 | 1 |
44 | France | 80.657 | 30470.01670 | 1 |
47 | Germany | 79.406 | 32170.37442 | 1 |
49 | Greece | 79.483 | 27538.41188 | 1 |
55 | Hong Kong, China | 82.208 | 39724.97867 | 1 |
57 | Iceland | 81.757 | 36180.78919 | 1 |
62 | Ireland | 78.885 | 40675.99635 | 1 |
63 | Israel | 80.745 | 25523.27710 | 1 |
64 | Italy | 80.546 | 28569.71970 | 1 |
66 | Japan | 82.603 | 31656.06806 | 1 |
71 | Kuwait | 77.588 | 47306.98978 | 1 |
90 | Netherlands | 79.762 | 36797.93332 | 1 |
91 | New Zealand | 80.204 | 25185.00911 | 1 |
95 | Norway | 80.196 | 49357.19017 | 1 |
113 | Singapore | 79.972 | 47143.17964 | 1 |
115 | Slovenia | 77.926 | 25768.25759 | 1 |
118 | Spain | 80.941 | 28821.06370 | 1 |
122 | Sweden | 80.884 | 33859.74835 | 1 |
123 | Switzerland | 81.701 | 37506.41907 | 1 |
125 | Taiwan | 78.400 | 28718.27684 | 1 |
133 | United Kingdom | 79.425 | 33203.26128 | 1 |
134 | United States | 78.242 | 42951.65309 | 1 |
data.query("label == 0")
country | life_exp | gdp_cap | label | |
---|---|---|---|---|
4 | Argentina | 75.320 | 12779.379640 | 0 |
13 | Botswana | 50.728 | 12569.851770 | 0 |
14 | Brazil | 72.390 | 9065.800825 | 0 |
15 | Bulgaria | 73.005 | 10680.792820 | 0 |
23 | Chile | 78.553 | 13171.638850 | 0 |
29 | Costa Rica | 78.782 | 9645.061420 | 0 |
31 | Croatia | 75.748 | 14619.222720 | 0 |
32 | Cuba | 78.273 | 8948.102923 | 0 |
33 | Czech Republic | 76.486 | 22833.308510 | 0 |
40 | Equatorial Guinea | 51.579 | 12154.089750 | 0 |
45 | Gabon | 56.735 | 13206.484520 | 0 |
56 | Hungary | 73.338 | 18008.944440 | 0 |
60 | Iran | 70.964 | 11605.714490 | 0 |
70 | Korea, Rep. | 78.623 | 23348.139730 | 0 |
72 | Lebanon | 71.993 | 10461.058680 | 0 |
75 | Libya | 73.952 | 12057.499280 | 0 |
78 | Malaysia | 74.241 | 12451.655800 | 0 |
81 | Mauritius | 72.801 | 10956.991120 | 0 |
82 | Mexico | 76.195 | 11977.574960 | 0 |
84 | Montenegro | 74.543 | 9253.896111 | 0 |
96 | Oman | 75.640 | 22316.192870 | 0 |
98 | Panama | 75.537 | 9809.185636 | 0 |
102 | Poland | 75.563 | 15389.924680 | 0 |
103 | Portugal | 78.098 | 20509.647770 | 0 |
104 | Puerto Rico | 78.746 | 19328.709010 | 0 |
106 | Romania | 72.476 | 10808.475610 | 0 |
109 | Saudi Arabia | 72.777 | 21654.831940 | 0 |
111 | Serbia | 74.002 | 9786.534714 | 0 |
114 | Slovak Republic | 74.663 | 18678.314350 | 0 |
117 | South Africa | 49.339 | 9269.657808 | 0 |
129 | Trinidad and Tobago | 69.819 | 18008.509240 | 0 |
131 | Turkey | 71.777 | 8458.276384 | 0 |
135 | Uruguay | 76.384 | 10611.462990 | 0 |
136 | Venezuela | 73.747 | 11415.805690 | 0 |
Another Example#
We can compress images using clustering by reducing the number of bytes.
from PIL import Image
import requests
url = "https://raw.githubusercontent.com/aoguedao/neural-computing-book/main/images/coyoya.jpg"
im_filapath = requests.get(url,stream=True).raw
im = Image.open(im_filapath)
im
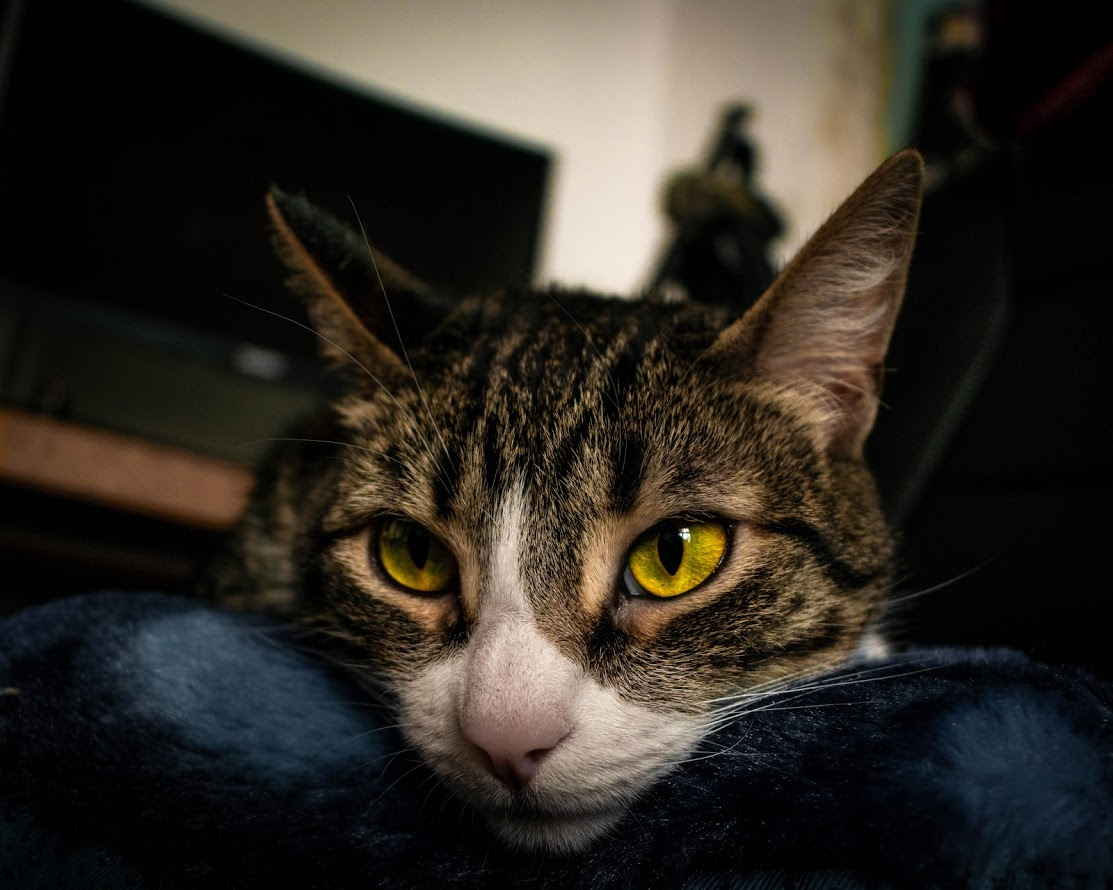
K = 8 # Number of clusters
X = np.array(im.getdata()) # Array with image values
kmeans = KMeans(n_clusters=K)
kmeans.fit(X)
compressed_array = kmeans.cluster_centers_[kmeans.predict(X)] # Prediction
im_compressed = compressed_array.astype(np.uint8).reshape(im.size[1], im.size[0], 3) # New image
Image.fromarray(im_compressed, mode="RGB")
/opt/hostedtoolcache/Python/3.8.16/x64/lib/python3.8/site-packages/sklearn/cluster/_kmeans.py:870: FutureWarning: The default value of `n_init` will change from 10 to 'auto' in 1.4. Set the value of `n_init` explicitly to suppress the warning
warnings.warn(
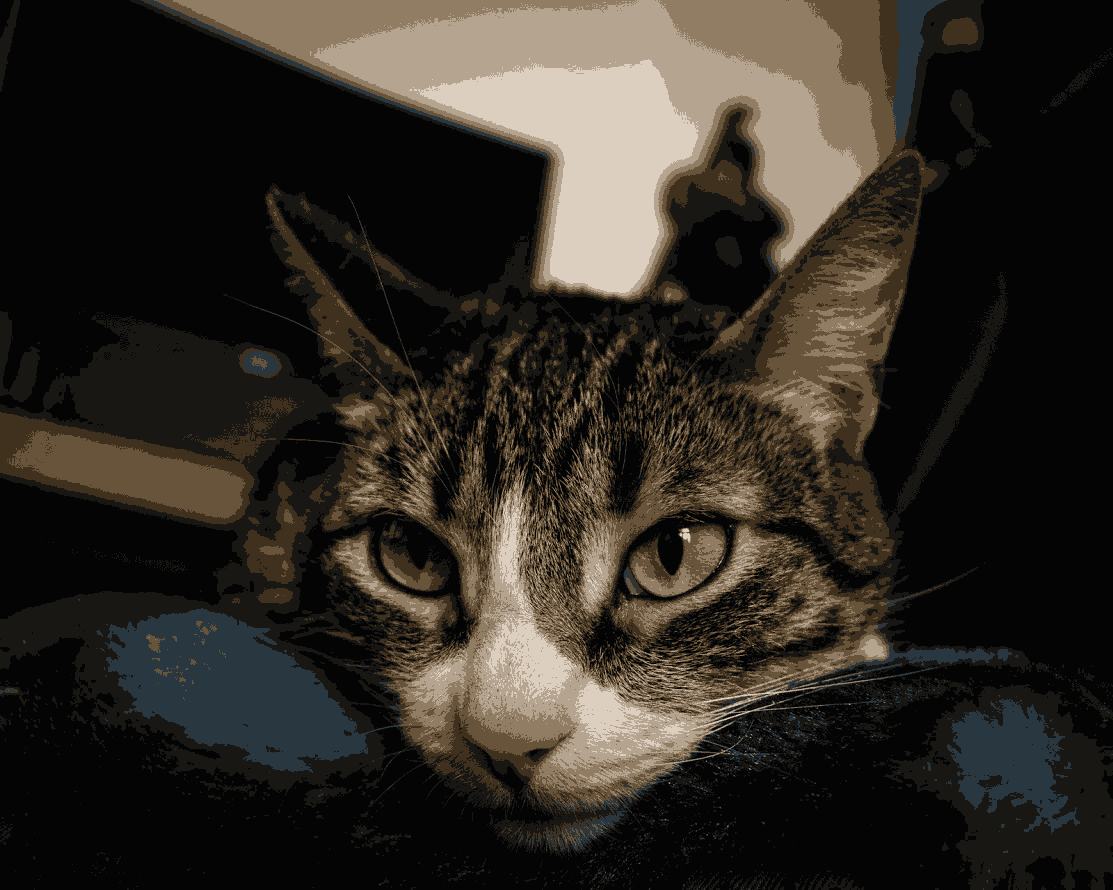