Activity - Data Visualization#
import pandas as pd
import matplotlib.pyplot as plt
import seaborn as sns
sns.set_theme(style="darkgrid")
We will use the breast cancer dataset for this activity. The main goal is to create visualization for finding the attributes that could predict or classify the type of tumor.
Attribute |
Domain |
---|---|
Sample code number |
id number |
Clump Thickness |
1 - 10 |
Uniformity of Cell Size |
1 - 10 |
Uniformity of Cell Shape |
1 - 10 |
Marginal Adhesion |
1 - 10 |
Single Epithelial Cell Size |
1 - 10 |
Bare Nuclei |
1 - 10 |
Bland Chromatin |
1 - 10 |
Normal Nucleoli |
1 - 10 |
Mitoses |
1 - 10 |
Class |
(2 for benign, 4 for malignant) |
More details here.
breast_cancer_data = pd.read_csv(
"https://archive.ics.uci.edu/ml/machine-learning-databases/breast-cancer-wisconsin/breast-cancer-wisconsin.data",
names=[
"code",
"clump_thickness",
"uniformity_cell_size",
"uniformity_cell_shape",
"marginal_adhesion",
"single_epithelial_cell_size",
"bare_nuclei",
"bland_chromatin",
"normal_cucleoli",
"mitoses",
"class",
],
index_col=0
)
breast_cancer_data.head()
clump_thickness | uniformity_cell_size | uniformity_cell_shape | marginal_adhesion | single_epithelial_cell_size | bare_nuclei | bland_chromatin | normal_cucleoli | mitoses | class | |
---|---|---|---|---|---|---|---|---|---|---|
code | ||||||||||
1000025 | 5 | 1 | 1 | 1 | 2 | 1 | 3 | 1 | 1 | 2 |
1002945 | 5 | 4 | 4 | 5 | 7 | 10 | 3 | 2 | 1 | 2 |
1015425 | 3 | 1 | 1 | 1 | 2 | 2 | 3 | 1 | 1 | 2 |
1016277 | 6 | 8 | 8 | 1 | 3 | 4 | 3 | 7 | 1 | 2 |
1017023 | 4 | 1 | 1 | 3 | 2 | 1 | 3 | 1 | 1 | 2 |
Just for this time we will drop the bare_nublei
column.
breast_cancer_data.dtypes
clump_thickness int64
uniformity_cell_size int64
uniformity_cell_shape int64
marginal_adhesion int64
single_epithelial_cell_size int64
bare_nuclei object
bland_chromatin int64
normal_cucleoli int64
mitoses int64
class int64
dtype: object
breast_cancer_data.drop(columns="bare_nuclei", inplace=True)
breast_cancer_data.dtypes
clump_thickness int64
uniformity_cell_size int64
uniformity_cell_shape int64
marginal_adhesion int64
single_epithelial_cell_size int64
bland_chromatin int64
normal_cucleoli int64
mitoses int64
class int64
dtype: object
And let’s add a categorical column with the type of tumor.
class_dict = {2: "benign", 4: "malignant"}
breast_cancer_data["class_name"] = breast_cancer_data["class"].map(class_dict)
breast_cancer_data.head()
clump_thickness | uniformity_cell_size | uniformity_cell_shape | marginal_adhesion | single_epithelial_cell_size | bland_chromatin | normal_cucleoli | mitoses | class | class_name | |
---|---|---|---|---|---|---|---|---|---|---|
code | ||||||||||
1000025 | 5 | 1 | 1 | 1 | 2 | 3 | 1 | 1 | 2 | benign |
1002945 | 5 | 4 | 4 | 5 | 7 | 3 | 2 | 1 | 2 | benign |
1015425 | 3 | 1 | 1 | 1 | 2 | 3 | 1 | 1 | 2 | benign |
1016277 | 6 | 8 | 8 | 1 | 3 | 3 | 7 | 1 | 2 | benign |
1017023 | 4 | 1 | 1 | 3 | 2 | 3 | 1 | 1 | 2 | benign |
For example,
sns.scatterplot(
data=breast_cancer_data,
x="clump_thickness",
y="uniformity_cell_size",
hue="class_name"
)
<Axes: xlabel='clump_thickness', ylabel='uniformity_cell_size'>
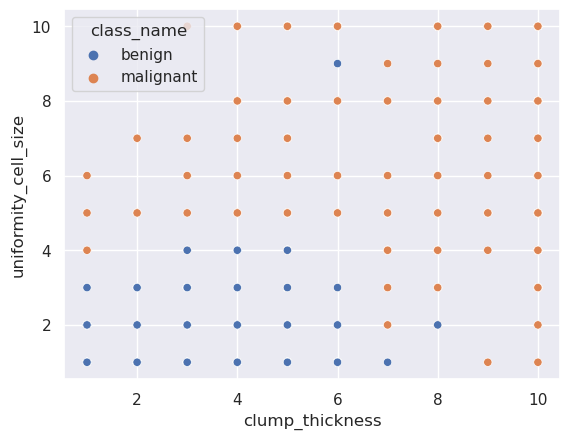
Your turn!